Crowdsensing: State of the Art and Privacy Aspects
1. Introduction
It is estimated that today approximately three-quarters of the human population has a mobile phone. Crowdsensing, a new business model, allows this large number of mobile phones to be used not only for exchanging information between their users, but also for activities that may have a huge societal impact.
The term "crowdsensing" refers to sharing data collected by sensing devices with the aim
to measure a phenomena of common interest. The sensing devices may include, for example, smartphones (Google Nexus, iPhone), sensor embedded gaming systems (XboX Kinect, Wii), music players (iPods), and in-vehicle sensor devices (OBD-II and GPS).
Crowdsensing applications are particularly attractive to organizations because they can provide the organizations with valuable data without the need to make significant investments. This is because tools like Mobile Campaign Designer simplify the creation of crowdsensing platforms. By using Mobile Campaign Designer, organizations can define the parameters of their crowdsensing campaigns, and the tool automatically generates the source code and an executable for a customized crowdsensing campaign. Even users without technical expertise are capable of creating a crowdsensing application for approximately five minutes.
The present article firstly examines the typology of crowdsensing (Section 2), the existing applications using crowdsensing (Section 3), and the privacy implications of crowdsensing (Section 4). Finally, a conclusion is drawn (Section 5).
2. Typology of Crowdsensing
Five categories of crowdsensing can be distinguished on the basis of two criteria. The criteria are (1) the involvement of the user in the crowdsensing process and (2) the type of the measured phenomenon. On the basis of the first criterion, we can distinguish participatory crowdsensing and opportunistic crowdsensing. In participatory crowdsensing, the users of the sensing devices actively send sensor data to a server. In opportunistic crowdsensing, the sending of information is automatic, with minimal involvement of the user.
On the basis of the second criterion, we can distinguish three types of crowdsensing: namely, environmental, infrastructure, and social crowdsensing. Environmental crowdsensing is used for measuring the natural environment (e.g., level of water, air pollution, wildfire habitats). Infrastructure crowdsensing is used for measuring the public infrastructure (e.g., traffic congestion and road conditions). The social crowdsensing is used for measuring data about the social life of individuals (e.g., the cinemas visited by an individual).
Table 1 shows the typology of crowdsensing described in this section.
Table 1: Typology of Crowdsensing
- Participatory crowdsensing
- Opportunistic crowdsensing
- Environmental crowdsensing
- Infrastructure crowdsensing
- Social crowdsensing
3. Existing Applications using Crowdsensing
Below, we examine the crowdsensing applications Creek Watch (Section 3.1), Nericell (Section 3.2), and DietSense (Section 3.3). These applications were chosen because they represent all of the aforementioned types of crowdsensing.
3.1 Creek Watch
Creek Watch is an iPhone application developed by IBM Almaden Research Center. The application monitors the water levels and the quality of the area around the water. Creek Watch allows the users of the application to submit the following information: (1) the amount of water they see; (2) the rate of flow; (3) the amount of trash they see; and (4) a picture of the waterway. The IBM Almaden Research Center aggregates the collected data and share it with institutions that are responsible for managing water resources. The data provided by the users is displayed on an interactive map. Fig.1 displays a screenshot of the interactive map used by IBM Almaden Research Center to display the contributions of the users. Because Creek Watch requires the users to submit environmental data manually, it uses participatory and environmental crowdsensing. The main incentive for using Creek Watch is the contribution to the environmental protection.
Fig. 1: A screenshot of the interactive map used by IBM Almaden Research Center to display the contributions of the users of Creek Watch
3.2 Nericell
The Nericell project is developed by Microsoft Research. The project allows people to automatically submit road traffic data by using their mobile smartphones. A person willing to participate in Nericell needs only to start an application installed on her smartphone. Then, the smartphone starts automatically monitoring road and traffic conditions and sends the collected information to the cloud for analysis. The road and traffic conditions are monitored through a range of sensors available on smartphones, such as accelerometer, Bluetooth, cellular radio, GPS, and microphone. For example, the microphone can be used to detect honking. The accelerometer can be used to detect potholes. Since Nericell automatically collects road traffic data from users, it uses opportunistic and infrastructure crowdsensing. The users participate in Nericell because the information submitted by them will facilitate road traffic.
3.3 DietSense
DietSense sense is a software system developed at UCLA (University of California, Los Angeles). DietSense allows individuals to share information about their eating habits with other individuals. Thus, the individuals can compare their eating habits. The individuals willing to use DietSense will need to respond to a survey requesting information such as photos of food, the motivation for choosing the food, and the place of preparation of the food. Individuals need to manually send the information about their eating habits. Therefore, DietSense uses participatory and social crowdsensing. The incentive for using DietSense is the improvement of eating habits.
4. The Privacy Implications of Crowdsensing
Most crowdsensing applications collect data from individuals which can be used for the identification of those individuals. Such data may include, for example, different tastes and interests of a particular user, as well as location data. It should be noted that opportunistic crowdsensing raises more privacy concerns than participatory crowdsensing. This is because the users of applications using opportunistic crowdsensing are not in a direct control of the data they submit. For example, the microphone can record not only noises from other cars, but also the voices of the passengers in the cars.
The creators of crowdsensing applications have to find a way to protect the data of individuals while at the same time enabling the operation of the applications. There are three main approaches on how to protect the privacy of the users of crowdsensing platforms. These approaches are anonymization (Section 4.1), encryption (Section 4.2), and data perturbation (Section 4.3).
4.1 Anonymization
Anonymization can be used to remove the identifying information collected by crowdsensing applications, but it raises two problems. Firstly, the mere removal of identifying information like names and addresses from the data cannot guarantee anonymity. For example, in cases where the crowdsensing applications collect location data, the anonymization will not be able to prevent the identification of individuals. The reason is that anonymized location data will still show the frequently visited locations of a person, which in turn may lead to the identification of that person. Secondly, in the context of data anonymization, data utility and data privacy are conflicting goals. As a result, the anonymization of data will enhance privacy protection, but decrease data utility.
4.2 Encryption
By encrypting data submitted by the users, unauthorized third parties will not be able to use personal data, even if they acquire access to the encrypted data. It should be noted that the encryption of a large volume of data may require significant computer resources.
4.3 Data Perturbation
Data perturbation refers to adding noise to sensor data immediately after the data is submitted by the individuals. As a result of the data perturbation, the data submitted by individuals will not be identifiable. Nevertheless, such data would enable good operation of crowdsensing applications.
One popular form of data perturbation is the micro-aggregation. The term micro-aggregation refers to replacing a specific field with an aggregate or more general value. The replacement of a ZIP code with the name of a province or a state is a typical example of micro-aggregation. Micro-aggregation can be operationally defined in terms of two steps, namely, partition and aggregation. Partition refers to partitioning the data set into several parts (groups, clusters). The aggregation refers to replacement of each record in a part with the average record.
5. Conclusion
Crowdsensing allows organizations to save significant financial resources because the users submitting the crowdsensing data are not paid. The users may have various incentives for submitting the data, such as avoiding traffic jams, preventing the consumption of not well-prepared food, and contributing to the protection of the environment. The submission of crowdsensing data does not require significant efforts by the users. Often, users need only to activate a crowdsensing application to start the automatic submission of data to a cloud. These characteristics of crowdsensing clearly indicate its huge potential.
In order to unleash the potential of crowdsensing, organizations need to have a better understanding of this relatively new concept. This article provided such an understanding by discussing a typology of crowdsensing and describing some of the existing crowdsensing applications. The typology may help the creators of crowdsensing applications to customize the crowdsensing processes in order to increase the effectiveness of the applications. The description of crowdsensing applications allows the readers to see how crowdsensing operates in practice.
Having explained the concept of crowdsensing and its practical application, the article next examined the privacy risks related to crowdsensing. It was pointed out that an adequate privacy protection of users of crowdsensing applications can be ensured only by the use of data perturbation. The disadvantages of the other methods for ensuring privacy protection outweigh the advantages.
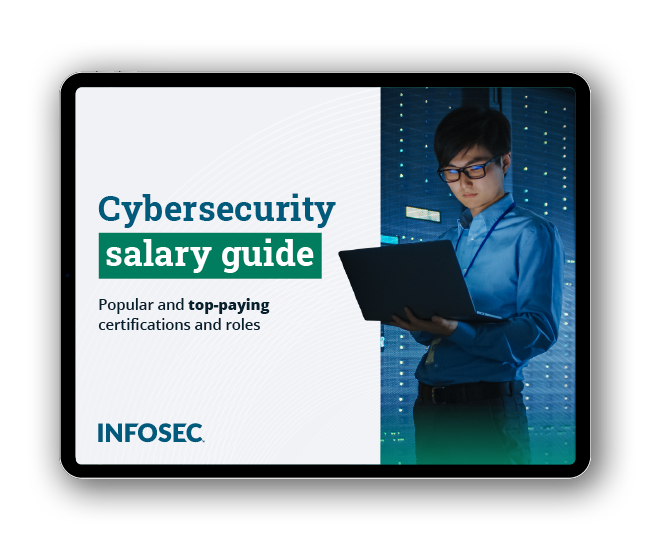
Get your guide to the top-paying certifications
With more than 448,000 U.S. cybersecurity job openings annually, get answers to all your cybersecurity salary questions with our free ebook!
References
- Baran, R., Galka, R., "CRM: The Foundation of Contemporary Marketing Strategy", Routledge, 2013.
- Byun, J., "Toward Privacy-preserving Database Management Systems --- Access Control and Data Anonymization", ProQuest, 2007.
- Foth, M., "From Social Butterfly to Engaged Citizen: Urban Informatics, Social, Media, Ubiquitous Computing, and Mobile Technology to Support Citizen Engagement", MIT Press, 2011.
- Ganti, R., Ye, F., Lei, H., "Mobile crowdsensing: current state and future challenges", IEEE Communications Magazine, Volume 49, Issue 11, November 2011.
-
Haderer, N., Rouvoy, R., Seinturier, L., "A preliminary investigation of user
incentives to leverage crowdsensing activities", Proceedings of Pervasive Computing and Communications Workshops (PERCOM Workshops), 2013 IEEE International Conference held on 18-22 March 2013.
- Heggen, S., Adagale, A., Payton, J., "Lowering the Barrier for Crowdsensing Application Development", Lecture Notes of the Institute for Computer Sciences, Social Informatics and Telecommunications Engineering Volume 130, 2014, pp 1-18.
- Qui, R., Hu, Z., Li, H., Wicks, M., "Cognitive Radio Communication and Networking: Principles and Practice", John Wiley & Sons, 2012.
- Ra, M., Liu, B., La Porta, T., Govindan, R., "Medusa: a programming framework for crowd-sensing applications", MobiSys '12 Proceedings of the 10th international conference on Mobile systems, applications and services, 2012.
- Rao, K., Bojkovic, Z., Bakmaz, B., "Wireless Multimedia Communication Systems: Design, Analysis, and Implementation", CRC Press, 2014.
- Sobh, T., Elleithy, K., "Emerging Trends in Computing, Informatics, Systems Sciences, and Engineering", Springer Science & Business Media, 2012.
- Solanas, A., González-Nicolás, U., Martínez-Ballesté, A., "Mixing Genetic Algorithms and V-MDAV to Protect Microdata", in "Computational Intelligence for Privacy and Security", Elizondo, D., (Ed.), Solanas, A., (Ed.), Martinez-Balleste, A., (Ed.).
- Stein, R., "Aligning Models and Data for Systemic Risk Analysis", in "Handbook on Systemic Risk", Fouque J. (Ed.), Langsam, J., (Ed.), Cambridge University Press, 2013.
- The official webpage of the project Nericell is available on http://research.microsoft.com/en-us/projects/nericell/ .
- The official webpage of the project Creek Watch is available on http://creekwatch.researchlabs.ibm.com/ .
- Zhao, F., Guibas, L., "Wireless Sensor Networks: An Information Processing Approach", Computers, 2004.