The Most Controversial Biometric of All – Facial Recognition
Overview of the Last Article
Our last article reviewed at length the latest Biometric modality to come, which is Vein Pattern Recognition, also known as VPR. It is probably the most widely accepted and used Biometric, for a number of key reasons:
- It is a non-contactless type of technology. Therefore, there is no direct, physical interaction required by the end user. Because of this, there are no hygiene issues associated with it, unlike Hand Geometry Recognition. It is very easy to deploy and implement, as well as training individuals on.
- It can be used in just about any kind of security related market application. For example, it can gamut the range from Physical Access Entry to Logical Access Entry to Time and Attendance, and even serve very effectively as a Multimodal Security based solution.
- The Vein Pattern amongst individuals is unique. Thus it also provides a fair amount of rich data which can be extracted.
- The Verification and/or Identification processing times are the quickest in the Biometrics Industry thus far. It is less than one second.
- Unlike Retinal Recognition, Iris Recognition, and Fingerprint Recognition, Vein Pattern Recognition is not prone to Civil Liberties or Privacy Rights issue. In other words, its social acceptance is the highest when compared to any other Biometric modality.
- It can capture the raw images and create the Enrollment and Verification Templates from two entirely different parts of the hand-the veins which exist in the fingertip and those which are present in the palm.
A key factor behind Vein Pattern Recognition is the level of deoxygenated blood which is carried in the vein structure throughout the entire human anatomy. Because of this, very robust images of the vein patterns can be captured. In fact, these raw images are deemed to be amongst the highest quality when compared to the other Biometric modalities.
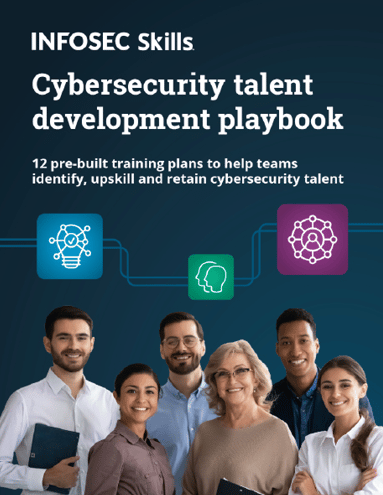
FREE role-guided training plans
As it was eluded to, Facial Recognition is very much prone to Civil Liberties and Privacy Rights Violations – thus, giving its appropriate title as the "most controversial Biometric of all."
Introduction to Facial Recognition
Just like Fingerprint Recognition, Facial Recognition is one of those Biometric modalities which everybody can relate to. For instance, we all have a face, and it continues to be used heavily to verify and confirm the identity of criminals and wanted suspects.
The best examples of this are the mug shots which are posted at the local post office and the websites of the federal law enforcement agencies.
The primary reason why Facial Recognition is prone to social phobias is that it can be used very covertly, without the slightest knowledge from the public. Probably of the best examples of this is the huge network of CCTV cameras which are deployed in London.
Although these are clearly visible to the naked eye, these cameras also possess Facial Recognition technology which can be used to confirm and verify the identity of any UK citizen without even a second thought being given to it by the public there.
Although Facial Recognition has come a long way since its first inception early in the last decade, it is still proving its worth as a highly reliable tool. This modality is very prone to the adverse effects of physical changes on the individual in question.
In other words, if a raw image is captured and the respective templates are created in which the individual is overweight in one instance, but then dramatically changes (such as massive weight loss) in the second instance, the system will not be able to confirm the identity of that same person.
Other variables which can impede a Facial Recognition system from conducting its Verification and/or Identification transaction processing are the addition/removal of any facial hair, and the aging process.
Also, the presence and absence of other factors such as hats, and the switching of glasses to contacts (and vice versa) can also have a negative impact on the performance of a Facial Recognition system.
But, the biggest advantage of Facial Recognition is that it can be used for very large scale Verification and/or Identification scenarios, such as that of the e-Passport infrastructure, and international law enforcement.
How Facial Recognition Works
Facial Recognition can be implemented either as a fully automated system or as a semi-automated system. With the former, no human intervention is required, but with the latter, some degree of it is mandatory. This is the preferred method to be used when deploying a Facial Recognition device.
This modality measures and extracts the distances of these prominent features of the face, from a central point (which can be at any part of the face)
- The ridges between the eyebrows
- The distances between the cheekbones
- The edges of the mouth
- The distances between the eyes
- The length and the width of the nose
- The contour and the profile of the jawline
- The length and the width of the chin.
To initiate the process of raw image collection, the individual must first stand in front of the Facial Recognition camera, to have multiple images of their face captured. These are then compiled into one master image, from which the unique features are then extracted.
However, before this can be done, the master image must either be aligned or further normalized in more granular detail.
Some of the techniques involved in this process include adjusting the face to be in the middle of the master image, or further tweaking the size and the angle of the face in question. These are all done via the use of specialized mathematical algorithms.
To help compensate for some of the obstacles as discussed in the last section, a method known as 3-D Imaging is utilized. With this, a shape of the face is created, from an already existing 2-D image of it. From here, the result is a facial model which can be applied to any 3-D plane.
Defining the True Effectiveness of a Facial Recognition System
Because of the degrees of unreliability which are still prevalent with Facial Recognition, the International Committee for Information Technology (also known as ("INCITS") has set forth some rather stringent requirements in order to guarantee the effectiveness of it. These are as follows
- The raw images of the face must include an entire composite of the head, and the individual must possess a full head of hair. Also, the raw images which are captured should also contain profiles of the neck and shoulders as well.
- The roll, pitch, and the yaw of the raw images must possess a variance of at least plus or minus 5 degrees of rotation.
- Only plain and diffused lighting should be used to capture the raw images of the face.
- Any kind of shadow which exists in the raw images must first be cropped out before the unique features can be extracted.
If 3-D imaging is used (as described in the last section), the following properties must be strictly observed
- Stereo imaging must use at least two different sets of cameras, and they should be mounted at a fixed distance.
- If structured lighting is used, the facial recognition system must then flash a well-defined, light beam onto the individual's face. This will then help to compute the various depth levels of the unique features of the face.
- Although laser scanners possess the most robust form of sensing, they should only be used on an as needed basis. The reasons for this is that they are very costly to implement, and are also very slow to capture and process the raw images of the face. For instance, it can take as long as 30 seconds to complete this process.
- Hybrid sensors should be favored over using laser scanners because it can use both stereo imaging and structured lighting in different combinations in order capture the best raw images possible.
It is also further mandated that the entire process of facial recognition must start with the location of the face in a set frame. To initiate the device, various cues and triggers can be implemented, such as the detection of the skin color, as well as the rotation and shape of the head.
Although these requirements have been implemented so that Facial Recognition can be on the same level of reliability as the other Biometric modalities, there are some serious limitations with these approaches. Examples of this include
- Identifying the differentiation between the tonality of the skin color and the background color in which the face appears in.
- Identifying the various shapes of the face (in this instance, Eigenfaces can be used this discussed in greater detail in the next section).
The Techniques of Facial Recognition
In order to foster an environment in which an image of the face can be detected in just one frame, various techniques have been developed, and can be grouped into two categories
- Appearance-based Facial Recognition Techniques
- Model-based Facial Recognition Techniques.
Appearance-based Facial Recognition Techniques
With this technique, a face can be represented in several distinct object views it can be based on just one image only, and no 3D models are utilized here. The two methodologies used in this category include PCA and Linear Discriminant Analysis (also known as "LDA").
PCA
This is a linear based technique, which dates all the way back to 1988 when Facial Recognition was first evolving. The concept of "Eigenfaces" is used here. These are simply 2-D spectral facial images which are composed of grayscale features. There are hundreds of Eigenfaces which can enter into the database of a Facial Recognition system.
When the raw images of the face are collected, this library of Eigenfaces is then superimposed over them. At this point, the level of variances between the Eigenfaces and the raw images are computed and averaged. Different statistical weights are then assigned.
The resultant of this process is a 1-Dimensional image of the face, which is further processed by the Facial Recognition system. In mathematical terms, the PCA is merely a linear transformation in which the raw images of the face get converted over into a geometrical coordinate system. To illustrate this, imagine a quadrant system.
The data set with the greatest statistical variance lies upon the first coordinate of the quadrant system (this is also termed as the "first PCA"), the next data set with the second largest statistical variance then falls onto the second coordinate. This process then continues in a descending fashion until a 1-D image of the face is created.
The biggest disadvantage with the PCA methodology is that it requires a full frontal image of the face. Thus, if there are any changes in the overall facial structure of the individual (such as weight loss or weight gain), then a full recalculation of the Eigenfaces is required.
Linear Discriminant Analysis (LDA)
With this methodology, the image of the face is projected onto a vector space. The primary objective of this is to speed up the Verification and/or Identification transaction processing times by drastically cutting down on the total number of facial features which need to be captured.
The mathematics behind the LDA methodology is to compute the level of variations that occur between a single raw data point and a single raw data record. Based on these specific calculations, the linear relationships are then extrapolated and formulated. By far, the strongest advantage of using this methodology is that it can take into consideration the lighting differences from the external environment.
After these linear relationships have been ascertained, the corresponding pixel values are captured, and statistically plotted. The resultant of this is a computed raw image of the face, which is also referred to as a "Fisher Face." To house these, an extremely large database is required, which can be a disadvantage.
Model-based Facial Recognition Techniques
Elastic Bunch Graph Matching (EBGM)
With this technique, the primary methodology utilized is that of Elastic Bunch Graph Matching (also known as "EBGM"). This examines the nonlinear mathematical relationships of the face, which includes such variables as the lighting differences in the external environment, as well as the variations in the facial expressions and poses of the individual.
To start the process, a facial map is first created. The image which is constructed on this map is just a sequencing of graphs, with various nodes located at the landmark features of the face. These include the eyes, the edges of the lips, tips of the nose, etc. These respective features then become 2-D distance based vectors, and Gabor mathematics are subsequently used to measure and calculate the variances of each vector on the facial image.
In the end, up to five spatial frequencies and up to eight different facial orientations can be formulated. One of the main advantages of using the EBGM methodology is that it does not require a full facial image, but the facial map must be created with great precision.
Conclusions
The evaluation of a Facial Recognition system can be broken down into the following categories
-
Universality
Unlike some of the other physical based Biometric modalities (such as Fingerprint Recognition and Hand Geometry Recognition), every individual has a face. So at least theoretically, everybody should be able to enroll into a Facial Recognition system.
-
Uniqueness
The face by itself is not distinctly unique at all. For example, members of the same family, as well as identical twins, share the same types of facial features. When it comes down to the DNA code, it is the overall facial structure which we inherit that contains the most resembling characteristics.
-
Permanence
The structure of the face can change greatly over the lifetime of an individual. As it was described earlier, the biggest factors affecting it are weight loss and weight gain, the aging process, as well as voluntary changes made to the face. As a result, it is quite likely that an individual will have to be enrolled over and over again into the Facial Recognition system to compensate for these variations.
-
Collectability
It can be quite difficult to extract the unique features of the face. This is primarily because any changes in the external environment can have a huge impact. For instance, the differences in the lighting, lighting angles, and the distance from which the raw images are captured can have a significant effect on the quality of the Enrollment and Verification Templates.
-
Acceptability
This is the category where Facial Recognition suffers the most. As it was described, it can be used covertly, thus greatly decreasing the public acceptance rate of it.
-
Resistance to circumvention
Unlike the other Biometric modalities, Facial Recognition systems can be very easily spoofed when 2-D models of the face are being used.
Sources
https://www.nyu.edu/projects/nissenbaum/papers/facial_recognition_report.pdf
http://www.face-rec.org/interesting-papers/general/imana4facrcg_lu.pdf
https://arxiv.org/ftp/arxiv/papers/1005/1005.4263.pdf
http://www.ehu.eus/ccwintco/uploads/e/eb/PFC-IonMarques.pdf
http://andrewsenior.com/papers/SeniorB02FaceChap.pdf
http://citeseerx.ist.psu.edu/viewdoc/download?doi=10.1.1.446.3828&rep=rep1&type=pdf
https://web.stanford.edu/class/ee368/Project_03/Project/reports/ee368group02.pdf
http://multimedia.3m.com/mws/media/833810O/facial-recognition-biometric-technology.pdf
http://wrap.warwick.ac.uk/47476/13/WRAP_rawlinson-bhalerao-wang-chap-2010.pdf
https://www.cs.ucsb.edu/~mturk/Papers/mturk-CVPR91.pdf
https://www.cs.ucsb.edu/~mturk/Papers/mturk-CVPR91.pdf
http://biometrics.nist.gov/cs_links/face/frvt/frvt2013/NIST_8009.pdf
http://www.eecs.umich.edu/courses/eecs487/w07/sa/pdf/nlydick-facial-recognition.pdf
http://cbcl.mit.edu/people/poggio/journals/brunelli-poggio-IEEE-PAMI-1993.pdf
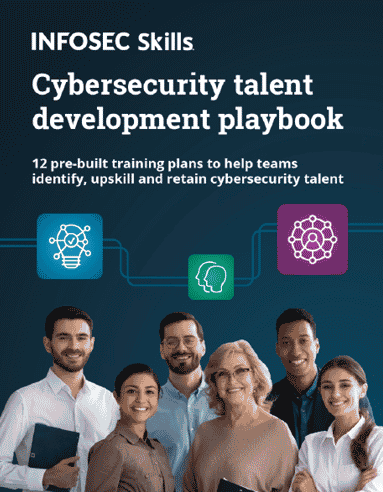
What should you learn next?